Smile Detection
Posed and spontaneous detection with wearable electromyography
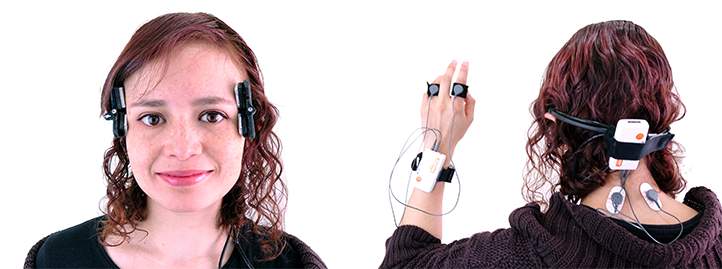
Facial expressions are among the most salient cues for automatic emotion identification. However, they do not always represent felt affect. As they are an indispensable social communication tool, they can also be fabricated to face complex situations in social interaction. In this work, identification of spontaneous and posed smiles was explored using multimodal wearable sensors.
Distal facial Electromyography (EMG) can be used to differentiate between different types of smiles robustly, unobtrusively, and with good temporal resolution. Furthermore, such wearable can be enhanced with autonomic electrophysiological and behavioral signals. Electrodermal Activity (EDA)-based recognition is a good indicator of affective arousal. Head movement is useful to capture the inherent movement during spontaneous expressions.
Result
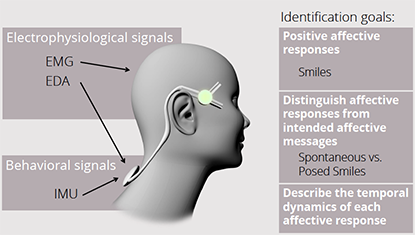
A multimodal wearable system was developed to continuously identify positive affective responses from electrophysiological and behavioral dynamics.
The focus is on the identification of positive cues of affect. These include smiles, skin conductance responses, and head movement.
Smiles were measured both from visible behavior through human coding of videos and computer vision; and from invisible behavior such as the muscle activity that generates the visually perceivable skin displacements.
Smiles are identified by an upward lifting of the lip corners, caused by activation of the Zygomaticus Major muscle. In the Facial Action Coding System (FACS), this movement is labelled as AU12. Although AU12 is the most characteristic feature of a smile, other facial movements often co-occur with it and are perceived as integral factors of these smiles. The Orbicularis Oculi (AU6, AU7), which raises the cheek and tightens the upper and lower eyelids, has also been described to be involved in felt smiles. This is the so-called Duchenne marker, named after Duchenne, who was the first to associate this movement with felt smiles during positive emotions. Nevertheless, recent studies have shown that the Duchenne marker is *not* an unequivocal marker of spontaneity.


Wearable facial distal EMG is a technique used to measure facial movement by placing the electrodes on the sides of the face. Thus, it does not obstruct facial movement, increasing ecological validity when compared to traditional EMG. It can be employed to broadly identify smile production, and to distinguish between different types of smiles, even when the mouth is covered:
- Smiles are detected with about 90% of accuracy, even when they are very quick and subtle.
- Spatial and magnitude feature analysis provided an accuracy of about 74% when distinguishing between posed and spontaneous smiles. On the other hand, using spatio-temporal features increased the accuracy to around 90% and reduced the inter-individual variability.
- Masking smiles, where a negative emotion is felt, but the smile is an attempt to conceal those feelings by appearing positive, are also distinguished from posed smiles with an accuracy over 90%, regardless of ethnic background.
- When compared to Computer Vision (CV), the mean accuracy of an intra-individual spatial features algorithm was 88% for CV, and 99% for EMG. With intra-individual spatio-temporal features, the mean accuracy was 87% for CV, and 91% for EMG. This suggests that EMG probably has the advantage of being able to identify covert behaviour that cannot be detected visually in intra-individual models.
Even though psychologists have asked similar questions in the past, none has addressed them with a multimodal wearable. This approach is a promising tool to explore with more temporal resolution how emotion processes arise and develop in our bodies. Moreover, this work has only proven the potential of the proposed approach. Future work should consider improving the wearable system for comfortable real-time usage in more ecologically valid settings.
Method
In four experiments, several research questions were asked and answered.
In Experiment 1, distal EMG was shown as an effective measure to identify fast and subtle spontaneous smiles, even at a micro-expression level. This is especially useful when two or more people are being tracked. Moreover, it was possible to identify the differences between posed and spontaneous smiles. Whilst the spatial distribution of the muscles differs, temporal features are more robust to distinguish among them. Namely, smile duration, rising time, and decaying time.


Experiment 2 confirmed the potential of using EMG to identify the smile's spatio-temporal dynamics. Special care was taken to elicit posed smiles intended to convey happiness. In this case, rising time and decaying speed differed significantly. EDA and IMU measures alone also have the potential to distinguish between co-occurring spontaneous and posed smiles with high accuracy. IMU-measured data explained best their differences. Moreover, no cultural differences were found between posed and spontaneous smiles from their embodied measures. These results seem to support the view that embodied affective responses are similar to all humans, regardless of their cultural background. Observed behavior was clearly related to the self-reported measures in both experiments.
Experiment 3 showed that laypersons can distinguish between posed and spontaneous smiles above chance level with modest accuracy. Hence, using behavioral and electrophysiological signals complements human ability, as they provide information not visually perceivable.
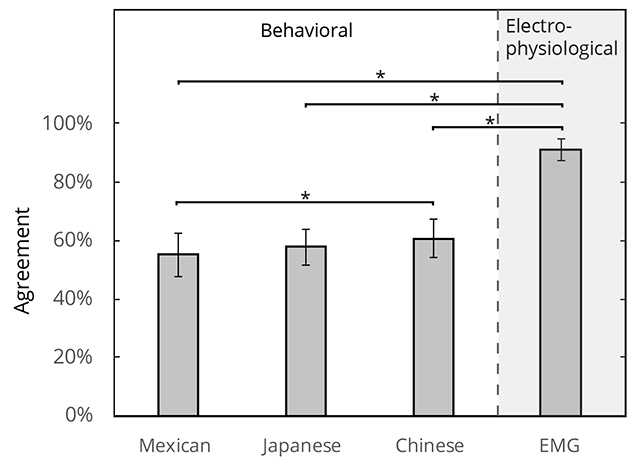
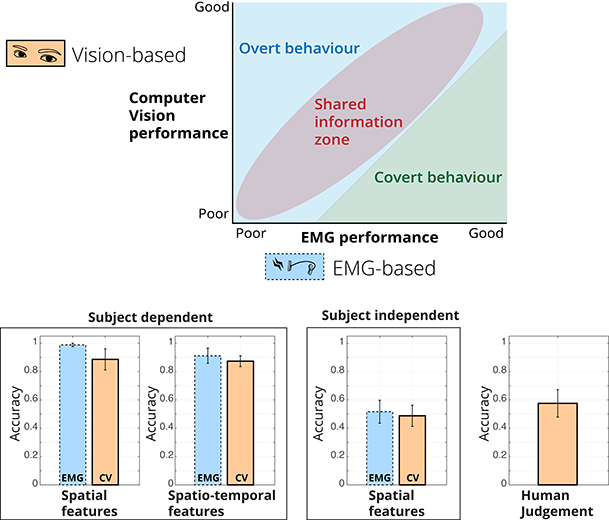
Experiment 4 was a data analysis test to unveil the extent to which non-visible behavior contributes to the successful identification of posed and spontaneous smiles. Using the data from experiment 2, analogous EMG-based and CV-based models were trained to distinguish the difference between posed and spontaneous smiles. The results showed that EMG has the advantage of being able to identify covert behavior not available through vision. On the other hand, CV appears to be able to identify visible dynamic features that human judges cannot account for. However, both technologies work best for individual-dependent models. Human judgement is still better than individual-independent models.
Resulting academic publications and presentations
Perusquía-Hernández, M., Ayabe-Kanamura, S., Suzuki, K., Human perception and biosignal-based identification of posed and spontaneous smiles. PLoS ONE 14(12): e0226328. 2019. DOI: 10.1371/journal.pone.0226328 | Download Cite |
Perusquía-Hernández, M., Hirokawa, M., Suzuki, K., A wearable device for fast and subtle spontaneous smile recognition. IEEE Transactions on Affective Computing Vol. 8, no. 4, pp. 522-533. 2017. DOI: 10.1109/TAFFC.2017.2755040 | Download Cite |
Perusquía-Hernández, M., Ayabe-Kanamura, S., Suzuki, K. “Affective Assessments with Skin Conductance Measured from the Neck and Head Movement”. Proceedings of the 8th Affective Computing and Intelligent Interaction Conference. 2019. DOI: 10.1109/ACII.2019.8925451 | Download Cite |
Perusquía-Hernández, M., Ayabe-Kanamura, S., Suzuki, K., and Kumano, S. “The Invisible Potential of Facial Electromyography: A Comparison of EMG and Computer Vision when Distinguishing Posed from Spontaneous Smiles”. In CHI Conference on Human Factors in Computing Systems Proceedings (CHI 2019), 9 pages. 2019. DOI: 10.1145/3290605.3300379 | Download Cite |
Perusquía-Hernández, M., Hirokawa, M., Suzuki, K., “Spontaneous and Posed Smile Recognition Based on Spatial and Temporal Patterns of Facial EMG”, Proceedings of the 7th Affective Computing and Intelligent Interaction Conference, pp. 537-541, 4 pages. 2017. | Download Cite |
Perusquía-Hernández, M., Suzuki, K., “A wearable device for fast and subtle spontaneous smile recognition”. Invited talk at the Nichibokubashi Symposium at the Mexican Consulate. 2017. |